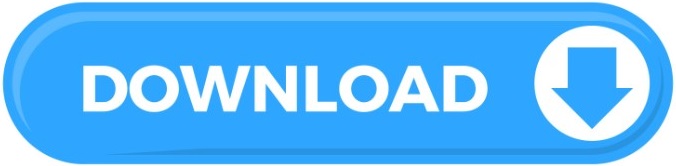
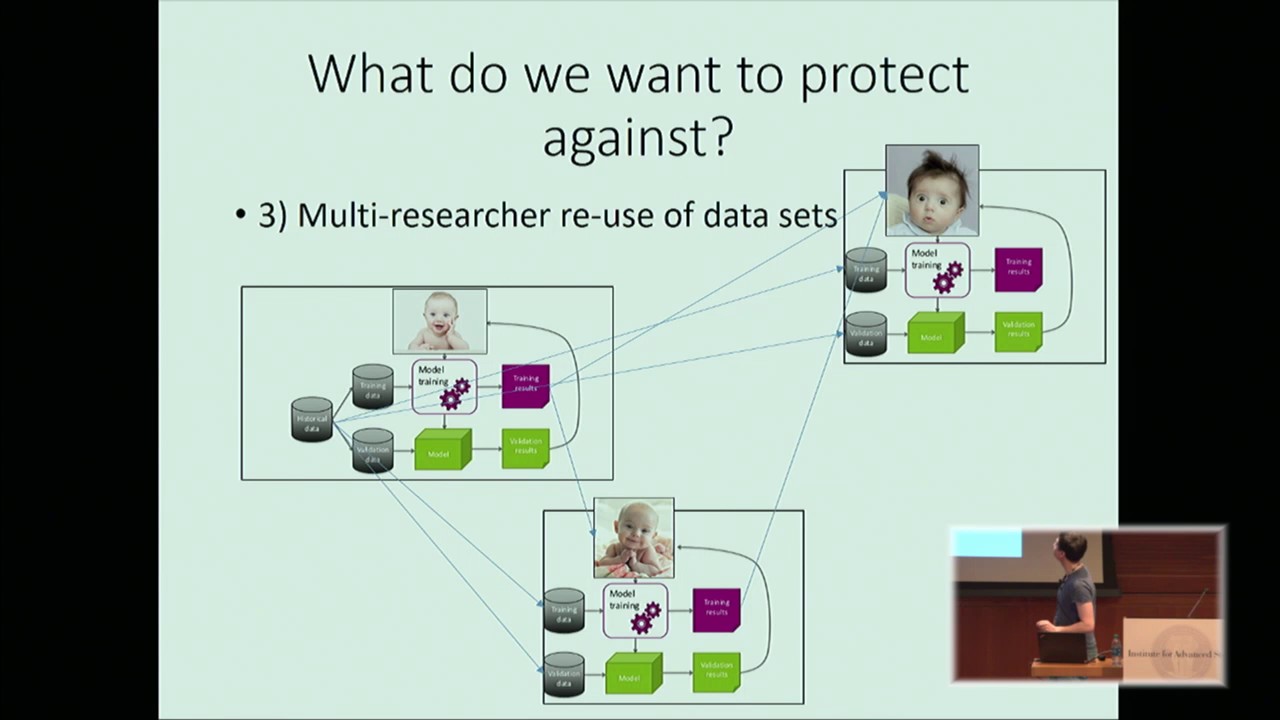
To analyze a large volume of data, it is imperative to study them carefully. This can be as extreme as the actual results, supposing that the null hypothesis is the correct observation. In null-hypothesis importance testing, the p-value is the probability of getting test results. This supposition is known as the null hypothesis, and H0 denotes it. A cautious hypothesis of the distribution or parameter is made. Hypothesis testing is a method of statistical assumption that takes data from a sample to conclude a population consideration or a distribution of population probability. P-Hacking could have been done by mistake or knowingly for any reason.īut how is this done? This is achieved by the performance of multiple data tests and then finding a pattern followed by modifying some of the values for having a biased result. P-hacking is misusing data analysis which shows that the data patterns are important statistically. There are risks that come along the way when the p-value is misused. So the null hypothesis is no longer valid. This means that the data supports the observed value more than they support the null value.īy standard rules, when the p-value is lower than 0.05, the result can be statistically important. Which is why the observed indicator is far from the null value.

If the p-value computation is a small value, then we can infer the tail that extends beyond the observed stats is small.
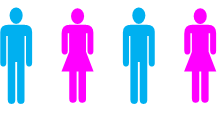
The test statistics are equal to the observed data value or It could also be more biased towards the p-value. P-value or probability value is the chance that depends on the null hypothesis model. It is difficult to avoid P-hacking but there are certain safeguards that may b help in reducing the chances of p-hacking and avoid the data dredging trap. It can also mislead other processes of data recording and computing an inference and result in an increased bias. However, it can severely impact the data by increasing the many false positives, which affects the study’s quality. P-hacking can also be described as an unintentional cherry-picking method of culling important and reliable data that leads to a surplus of noteworthy and required results. But why is this concerning? Because it affects the study of data in negative ways. Data dredging is extremely tough to spot. P-hacking is a way in which data analytics is exploited to find trends that seem statistically important but are not really important.
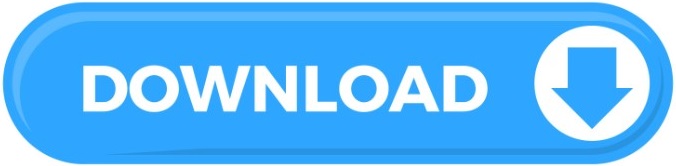